Key Technologies
At Qlector, we bring together advanced technologies and a deep understanding of shop-floor challenges to help manufacturers better plan, guide, and optimize their production.
Working behind the scenes, these technologies convert complex data into clear, actionable insights and informed decisions.
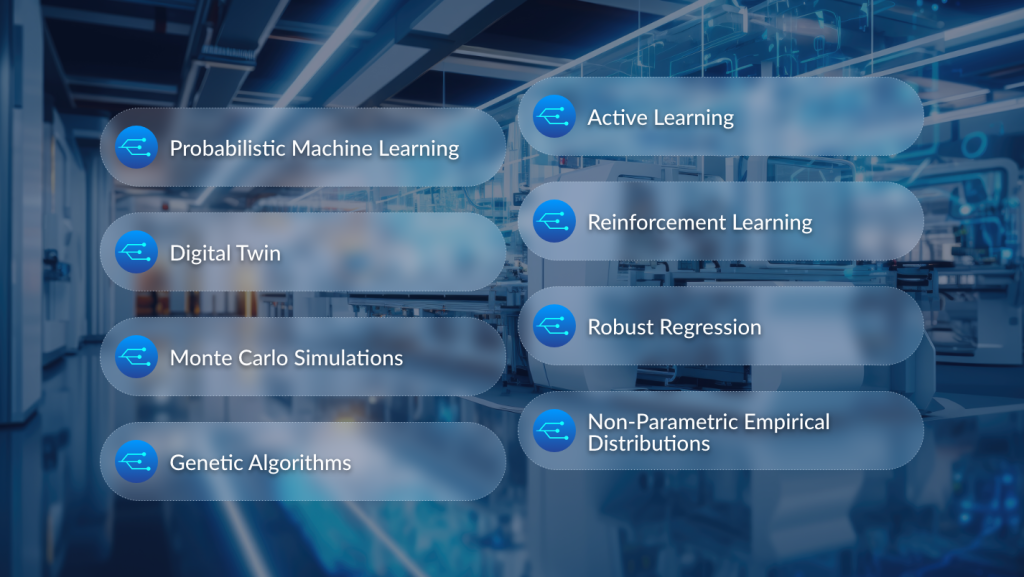
Since 2015, in-house research and development has produced secure, reliable, and proven technologies that power Qlector solutions.Discover more about how it all started.
Since 2015, in-house research and development has produced secure, reliable, and proven technologies that power Qlector solutions.Discover more about how it all started.
Technology Overview
The Building Blocks of Qlector LEAP
Probabilistic Machine Learning
Probabilistic machine learning is a method that considers different possible outcomes instead of just one definite result. It works by looking at various scenarios and assigning each a likelihood. This allows the system to understand many factors that affect a decision, allowing better predictions even when conditions change.
In manufacturing, probabilistic machine learning enables more accurate forecasting, even when data is incomplete or highly variable. For instance, the time needed to complete an order is influenced by many factors – such as machine availability, material supply fluctuations, or worker experience. Probabilistic models incorporate all these uncertainties to help planners make better-informed decisions, that boost efficiency and minimize surprises.
Digital Twin
A digital twin is a real-time virtual replica of a physical system. It continuously updates with data from the real world, creating a dynamic model that mirrors material flows, tool changes, workforce schedules, and machine statuses. This approach helps visualize and simulate the behavior of the system in a simplified digital environment.
In manufacturing, a digital twin serves as a smart map of production processes and material flows. It quickly spots potential disruptions like imbalanced workloads or material shortages and allows testing of different strategies before changes are made in the real world. This proactive monitoring helps optimize processes and minimizes downtime.
Monte Carlo
Monte Carlo simulations are a method that uses random sampling to explore a wide range of possible outcomes. Instead of calculating one fixed result, the system runs many repeated trials to generate realistic scenarios. This approach helps to capture uncertainty and variability in complex systems, offering a broad perspective on what might happen.
In manufacturing, these simulations help answer questions such as: “What’s the probability that this order will be completed on time?” By modeling thousands of possible outcomes—considering machine breakdowns, supply delays, or variations in worker efficiency— AI-based APS systems can provide planners with a clearer view of potential risks and uncertainties.
Non-Parametric
Non-Parametric Empirical Distributions build models directly from observed data without forcing it into a specific shape, like a bell curve. Traditional approaches often assume data follows a familiar pattern, but in many cases— especially in real-life scenarios—data behaves differently. This technique allows the model to learn from historical data as it is, capturing patterns such as multiple peaks or long tails, which represent more complex behavior.
In manufacturing, using non-parametric empirical distributions means working with data that reflects the true nature of the production process. This approach enables AI-based APS systems to detect anomalies, predict downtime, and identify potential bottlenecks more accurately by relying on realistic data patterns.
Genetic Algorithms
Genetic algorithms are inspired by natural evolution. They work by creating a variety of potential solutions and then selecting and combining the best ones through processes similar to biological reproduction and mutation. Over multiple iterations, the algorithm refines these solutions, gradually improving them without having to test every single possibility.
In manufacturing, genetic algorithms are often used for scheduling and resource allocation. Instead of testing every possible arrangement, they use a “survival of the fittest” approach to develop efficient production plans. This method helps optimize machine use, align worker shifts, and reduce downtime, ensuring a smoother and more effective manufacturing process at Qlector.
Active Learning
Active learning is a machine learning approach where the model actively selects the most informative data to learn from, often reaching out to human experts when it faces uncertain cases. Instead of passively processing all available data, the system focuses on ambiguous or challenging examples, asking for expert input to refine its understanding and improve prediction accuracy over time.
In manufacturing, active learning enhances automated systems by incorporating operator feedback. For instance, when the system detects unusual patterns or uncertain conditions in production, it prompts experts to provide their insights. This human-machine collaboration helps uncover issues that data alone might miss, leading to more reliable forecasts and smoother operations.
Reinforcement learning
Reinforcement learning is a type of machine learning where a system learns to make decisions through trial and error. It takes actions in an environment and receives feedback in the form of rewards or penalties, gradually learning which actions lead to the best outcomes.
In manufacturing, reinforcement learning continuously monitors production processes and tests different actions to improve efficiency. For example, it might identify and address bottlenecks by suggesting operational changes and then learning from the results. This ongoing process of adjustment and improvement helps optimize production schedules and resource allocation.
Robust Regression
Robust regression is a modeling technique that remains accurate even when the data includes errors, outliers, or unusual values. Unlike standard methods that can be strongly influenced by extreme data points, robust regression identifies these anomalies and reduces their impact. This makes the model more stable and reliable, especially when working with messy or imperfect data.
In manufacturing, data is often affected by things like sensor faults, manual input errors, or rare disruptions. Robust regression helps Qlector’s system stay focused on the usual patterns in production, instead of being misled by occasional mistakes or irregular events. As a result, it supports more consistent forecasting and planning, even in complex or noisy environments.
PROVEN TECHNOLOGY, TRUSTED BY
SCIENTIFIC LECTURES
Watch scientific lectures from
our team
Marko Grobelnik | Consulting OECD for AI
Limits of the current state of Artificial Intelligence for Law
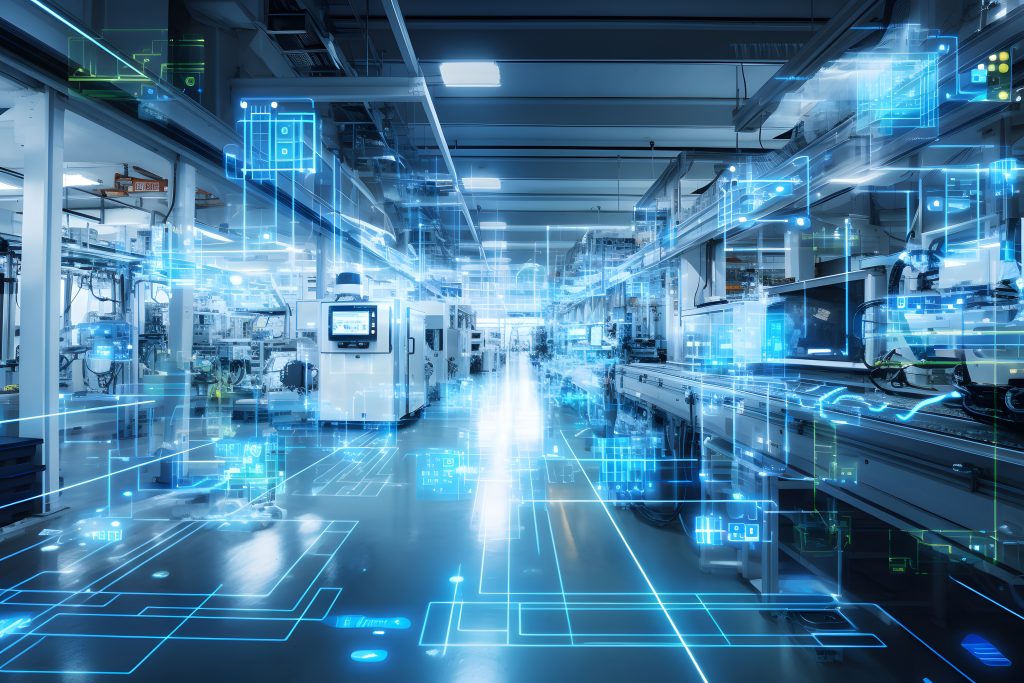
Security First
The Company implements a holistic information security management system (ISMS), based on the ISO 27001:2013 standard. The system provides a framework for continuous development, improvement, and education of all employees of the Company with the aim to perform their activities in line with security goals.
Ready to solve your planning challenges?
Talk to an expert and explore the potential of Qlector LEAP on your shop floor.