Have you ever wondered what AI projects in manufacturing really look like?
If you’ve been involved in AI projects on the shop floor, you know that getting quality data ready takes a lot of time.
In fact, only about 10 – 20% of the project goes into building the model, while the remaining 80 – 90% is all about collecting, cleaning, and preparing data.
This was one of the key insights at the Smart Manufacturing Forum held this March in Ljubljana.
At Qlector, we were proud to co-organize and sponsor the event alongside the German-Slovene Chamber of Commerce and Industry (AHK Slowenien) and Siemens, where industry experts, innovators, and decision-makers came together to share real-life experiences, best practices for SMEs, and insights from top manufacturers.
A huge thank you to everyone who brought their energy and curiosity to the event!
So, what were the key insights from the event? What did we learn?
No Data – No AI
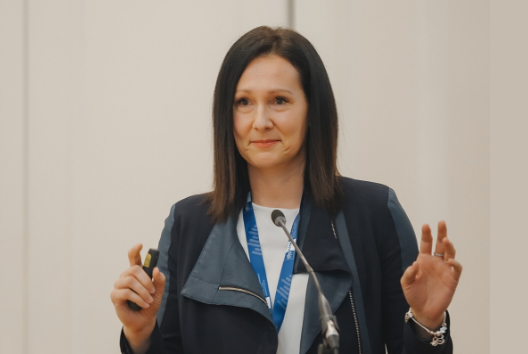
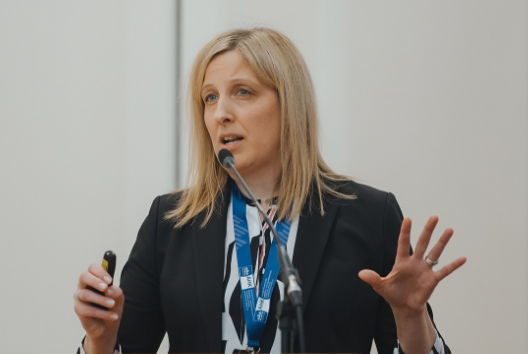
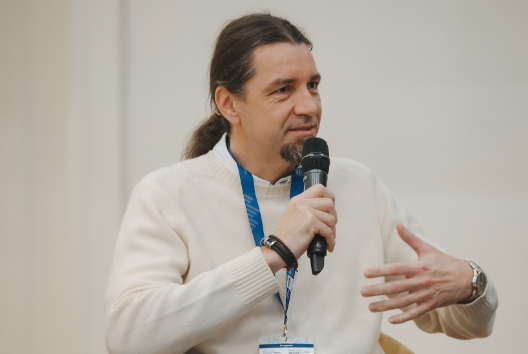
Adopting smart technology can offer a competitive advantage, but the true benefits arise only when the system delivers as expected.
As our CEO, Mateja Lavric, pointed out, these benefits materialize when there is quality data to work with.
Our CPO, Viktor Jovanoski, agreed with Mateja that one of the biggest challenges on the shop floor is the lack of good data and, as industry trends suggest, a shortage of skilled professionals.
For example, the Advanced Manufacturing Report stated that 98% of manufacturing leaders face at least one data-related challenge, while 35% struggle to derive actionable insights from their data.
This highlights the importance of investing in a strong data infrastructure and developing the knowledge needed to bridge the gap between technical expertise and business understanding.
When manufacturers meet the data prerequisites and properly implement smart technology in their production plants, they can experience benefits such as time savings for employees and improvements in key performance indicators (KPIs).
Nina Bolko, Supply Chain Manager at Kolektor, revealed that the implementation of the AI-powered solution Qlector LEAP significantly reduces the daily workload of both their MRP Planner and Production Team Leader, while also enhancing delivery accuracy and optimizing inventory levels for semi-finished parts and work-in-progress.
Speaking about the value their colleagues get from Qlector LEAP, Nina mentioned that a Team Manager at Kolektor said, “Qlector LEAP is the most useful tool for me at Kolektor in recent years.”
Digital Twins Are Taking Off
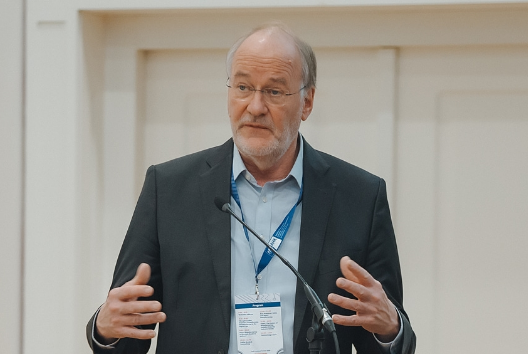
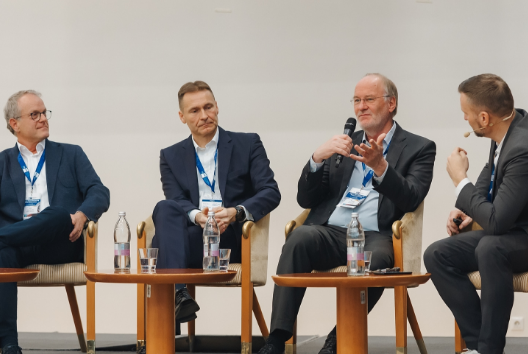
Dr. Harald Schöning, Vice President of Research at Software AG, emphasized the importance of understanding the current state of every machine and product.
He stressed that having a digital representation—or digital twin—of these elements, and even combining them, provides a real-time view of the production system and supports what-if simulations.
As Rok Koren, Senior Digitalization Consultant at Siemens d.o.o, stated, real-time data and digital twins are no longer optional—they are essential.
And this is not unexpected, considering their popularity among manufacturers.
McKinsey’s 2022 survey of senior executives in industrial sectors found that 86% of respondents believed a digital twin was applicable to their organization. Additionally, 44% had already implemented a digital twin, and 15% were planning to deploy one.
Furthermore, Digital Twin Market Report 2023–2027 stated that 29% of global manufacturing companies have either fully or partially implemented their digital twin strategies.
But remember, digital twins only work well when the data they use is reliable.
Poor data quality can lead to wrong predictions, inaccurate schedules, unnecessary downtime, wasted resources, and higher costs.
When managers see a disconnect between what the digital twin shows and what actually happens, it can shake their trust in the technology.
What comes next? Industrial metaverse!
Building on this foundation, one of the highlights at the conference was a discussion about the future trend of the industrial metaverse, which connects separate digital twins into one unified system.
It’s like an interactive simulation where manufacturers can test different production scenarios, analyze real-time data, and allow employees to collaborate in a shared space to fine-tune operations, predict system failures, and avoid costly mistakes.
Essentially, they can see, analyze, and optimize everything in a virtual environment before making real-world changes.
Investing in the Lifeblood of Your Digital Twin
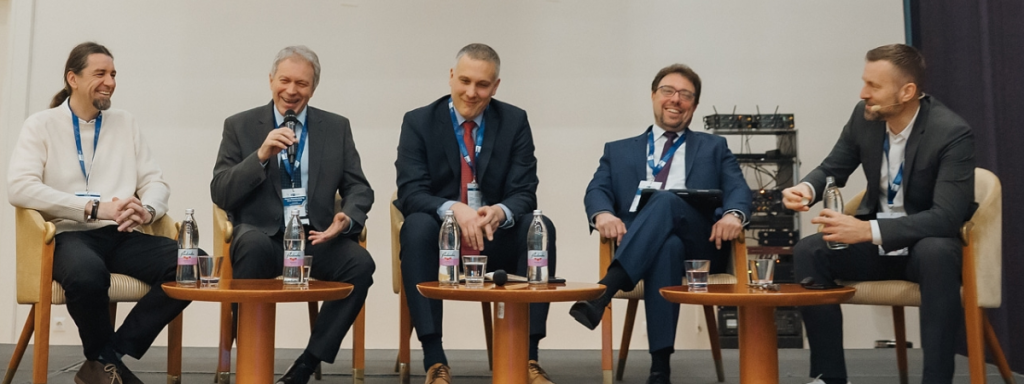
By putting in place strong data governance, validating data in real-time, and leveraging advanced analytics to cut through the noise, you’re not only avoiding risks but also building a solid foundation for success.
The better your data, the more vibrant and reliable your virtual counterpart becomes—and in today’s fast-paced shop floor, that’s one advantage that keeps you ahead of the curve.
So, what are your thoughts about this? How do you balance the time spent on preparing data versus developing models in your own AI projects?
Let’s discuss further 👇
Photo Credit: AHK Slovenija | Marko Delbello Ocepek