At the Global Smart Manufacturing Summit, Bosch Rexroth also delivered a lecture on managing material flow in battery gigafactories.
In May, I had the opportunity to attend the 9th Edition of the Global Smart Manufacturing Summit held in Berlin. The summit brought together industry leaders and innovators to discuss the future of manufacturing in the era of digital transformation. Inspired by the insights from the event, I am now taking the opportunity to explore and explain how these concepts can help you successfully and swiftly deploy AI in your manufacturing processes.
Key Takeaways
Presentations that stood out in Berlin addressed relevant topics in manufacturing, such as Data Value Stream, E2E Traceability, Strategies for Effective Digital Transformation, and Implementation of (Gen)AI. All the above-mentioned presentations can be summarized into seven key takeaways for effectively managing data and implementing AI technology in the manufacturing sector:
- Latest Trend: The Shift Toward Flexibility and Resilience
- Comprehensive AI Use Cases
- Overcoming Challenges in AI Implementation
- The Importance of a Robust AI Foundation
- Strategic Development and Scaling AI
- Change Management & People Enablement
- Steps for Immediate AI Implementation.
Harness the Benefits, Avoid the Mistakes
Based on the insights from Berlin and vast experience in over 40 implementations of our AI solution in manufacturing, let’s deep dive into the main takeaways and point out the key benefits of implementing AI while avoiding common mistakes and pitfalls.
Latest Trend: The Shift Toward Flexibility and Resilience
The imperative in digital manufacturing has evolved significantly, transitioning from a focus solely on costs and efficiency improvements to prioritizing enhanced flexibility and resilience. This shift is even more crucial in the VUCA (Volatile, Uncertain, Complex, Ambiguous) world we live in today. It prepares companies to handle unexpected market and environmental changes, thus aligning their AI strategies with broader business objectives.
Source: PwC Digital Factory Transformation Survey 2022, GenAI in Manufacturing presentation by Michael Bruns, PwC
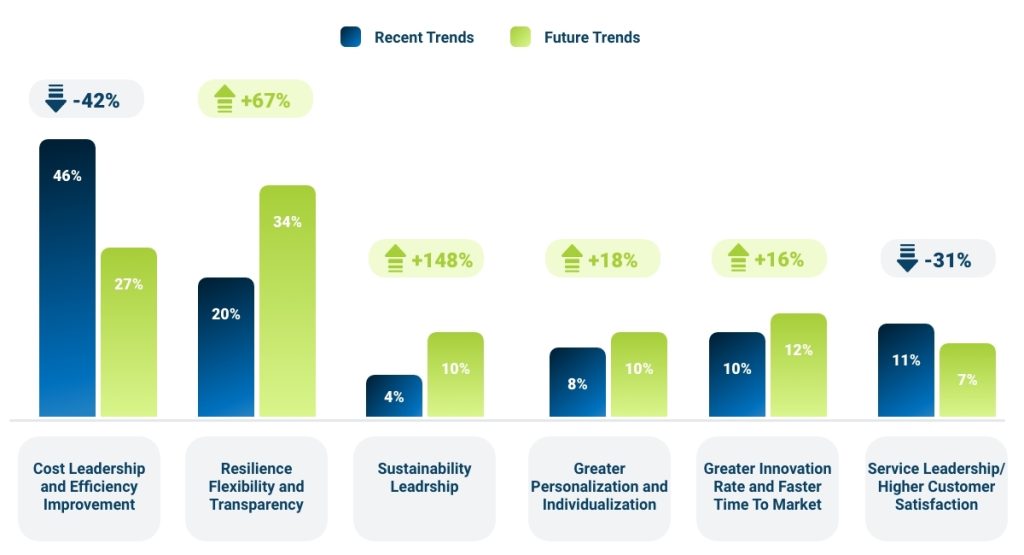
Action Steps
- Evaluate and Adapt: Regularly evaluate your manufacturing processes and operational strategies to ensure they can quickly adapt to new challenges and changes. Invest in new technologies that enhance flexibility.
- Ensure Dynamic Response: Utilize AI to predict potential disruptions, optimize supply chain operations, and maintain continuity in production under varying conditions.
Comprehensive (Gen)AI Use Cases
AI is impacting functions across the entire manufacturing value chain – from product and service development to planning, order management, and final delivery. Key applications include optimizing production schedules in real-time, balancing production lines end-to-end to avoid bottlenecks, and planning the supply chain based on real-time changes and constraints, along with real-time data monitoring to detect anomalies, among others (see infographic). These applications ensure that manufacturing is not only more efficient but also more adaptable to changing market demands and production dynamics.
Action Steps
- Thorough Analysis: Conduct a comprehensive analysis of your entire manufacturing value chain to identify potential areas for AI integration. Focus on stages that require enhancement in efficiency, quality, or safety.
- Drive Product and Process Innovation: Utilize generative design to enhance product features and customization, which can lead to a faster time to market and meet specific consumer demands.
- Optimize Production and Supply Chain: Implement AI solutions like production scheduling assistants and supply chain planning to streamline operations and reduce bottlenecks.
Overcoming Challenges in AI Implementation
Implementing AI presents several challenges that can hinder successful integration and optimal performance. These challenges include poor data quality, which can significantly undermine the effectiveness of (Gen)AI models, and integration complexity, where the vast and varied manufacturing systems pose significant hurdles. Additionally, organizational readiness and cultural resistance often slow down AI adoption, and gaps in necessary skills and knowledge can prevent effective utilization of AI at scale.
Action Steps
- Enhance Data Management: Focus on improving the quality of your data and implement robust data governance frameworks to ensure data accuracy, consistency, and security.
- Simplify Integration Processes: Develop standardized protocols and modular systems that simplify the integration.
- Boost Organizational Readiness: Cultivate an AI-positive culture within your organization by clearly communicating the benefits and transformative potential of AI. Involve key stakeholders early in the decision-making process.
- Close Skill and Knowledge Gaps: Invest in comprehensive training programs and partnerships with educational institutions to develop the necessary AI and data analytics skills within your workforce.
The Importance of a Robust AI Foundation
A well-designed (Gen)AI infrastructure is essential for reducing operational costs and accelerating the generation of actionable insights for data-driven decision making. This step involves creating an integrated platform architecture that ensures interoperability and scalability and supports various AI functions and data flows.
Action Steps
- Invest in Scalable Infrastructure: Build or enhance your data infrastructure to support comprehensive AI capabilities, focusing on scalability to handle future growth.
- Prioritize High-Quality Data: Develop data governance practices to ensure that the data fueling your AI systems is accurate, consistent, and secure.
- Assess Integration Capabilities: Regularly review your current IT infrastructure to identify and address potential integration challenges, ensuring smooth operation across different systems.
- Foster AI Literacy: Conduct training sessions and workshops to ensure that your team not only understands AI capabilities but also stays updated with the latest AI practices.
Strategic Development and Scaling AI
Scaling AI is not just about deploying technology – it’s about strategically developing and integrating AI to ensure it aligns with business goals and scales effectively. This requires a holistic approach to understanding AI use cases across the organization, ensuring these cases are scalable and have the necessary support structures in place, such as an integrated platform architecture and a well-defined organizational setup. The successful scaling of AI also depends on creating and maintaining a clear data strategy that focuses on business impact, ensuring data quality and accessibility.
Action Steps
- Develop a Roadmap: Include milestones and metrics for AI implementation success.
- Ensure Interoperability: Build systems that integrate seamlessly with existing technologies.
- Performance Monitoring: Continuously track AI against KPIs.
- Regular Updates: Assess and scale AI systems to meet evolving business needs and technological advancements.
Change Management & People Enablement
Effective change management and people enablement are essential for the successful adoption of (Gen)AI in manufacturing. This involves clear communication, stakeholder management, ongoing training to prepare employees for new technologies, and an open innovation organizational culture.
Action Steps
- Engage and Explain: Involve everyone in the organization, explaining the changes and their benefits clearly.
- Stakeholder Management: Assess and address stakeholders’ concerns and expectations regularly.
- Continuous Training: Provide ongoing educational programs that equip employees with necessary AI skills.
Practical Steps for Immediate AI Implementation
Implementing AI swiftly and effectively requires an approach that emphasizes data quality, strategic alignment with business goals, and agile development methods. To ensure that AI initiatives drive substantial business value, it’s crucial to develop a robust data strategy that covers collection, storage, analysis, and compliance with regulations.
Action Steps
- Audit Data Management: Review your current data management practices to ensure they support effective AI applications.
- Impactful KPIs: Establish clear KPIs that (Gen)AI solutions aim to enhance, providing measurable targets for success.
- High-Impact Use Cases: Focus on use cases that promise significant returns and align closely with strategic business objectives.
- Start Small and Scale: Kick off with small, manageable AI projects to secure quick wins, building organizational support and momentum.
- Agile Development: Employ iterative development methods to allow for rapid iteration and scaling based on user feedback and evolving business needs.
Resilience is about expecting the unexpected
For managers in the manufacturing sector, embracing (Gen)AI significantly enhances operational flexibility and resilience. By following these practical steps, one can not only optimize current processes but also future-proof operations in today’s VUCA (Volatile, Uncertain, Complex, Ambiguous) world.
Participating in the Global Smart Manufacturing Summit has undoubtedly deepened my understanding of the pivotal role AI technology plays in modern manufacturing. Let me know what your thoughts on the topic and proposed action steps are at [email protected].
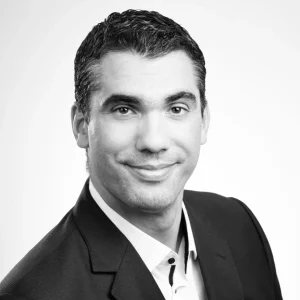
Ognjen Backović
Senior Business Development Expert